前言
這篇的摘要寫說針對AlexNet的基礎上主要做了兩方面的改進:
1.使用了最小的3x3卷積核尺寸和最小間隔。
- 在整個圖片和multi-scale上訓練和測試圖片。
論文原句:1. Use smaller receptive window size and smaller stride of the first convolutional layer.
2.Training and testing the networks densely over the whole image and over multiple scales.
|
|
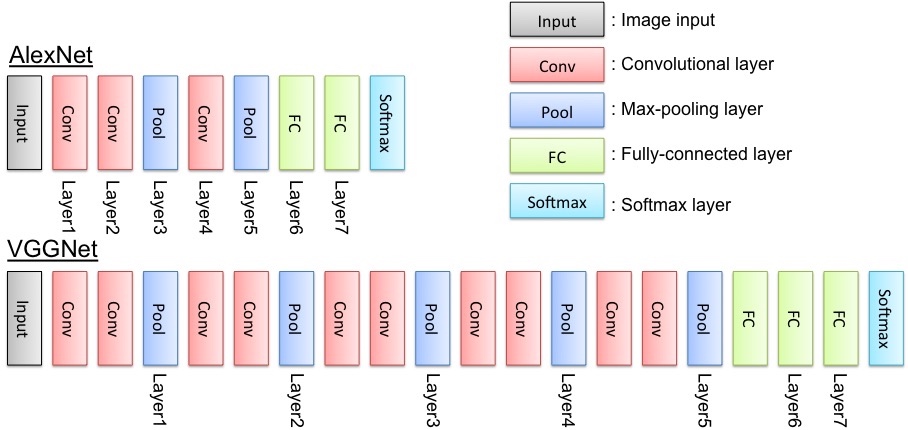
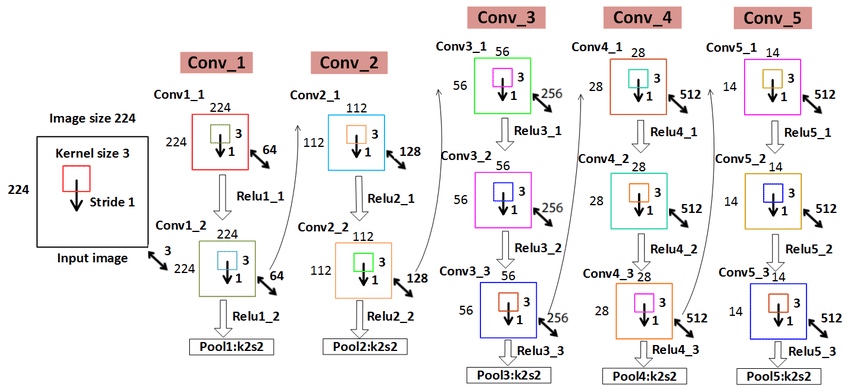
架構
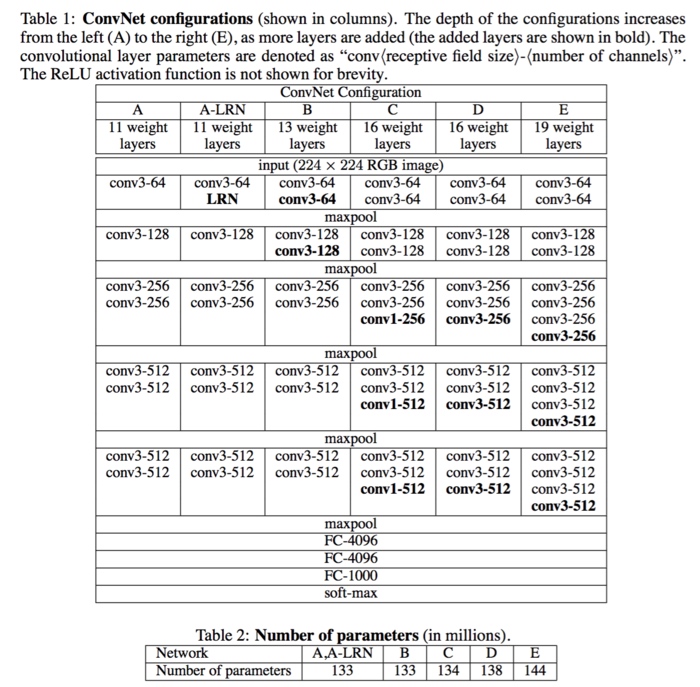
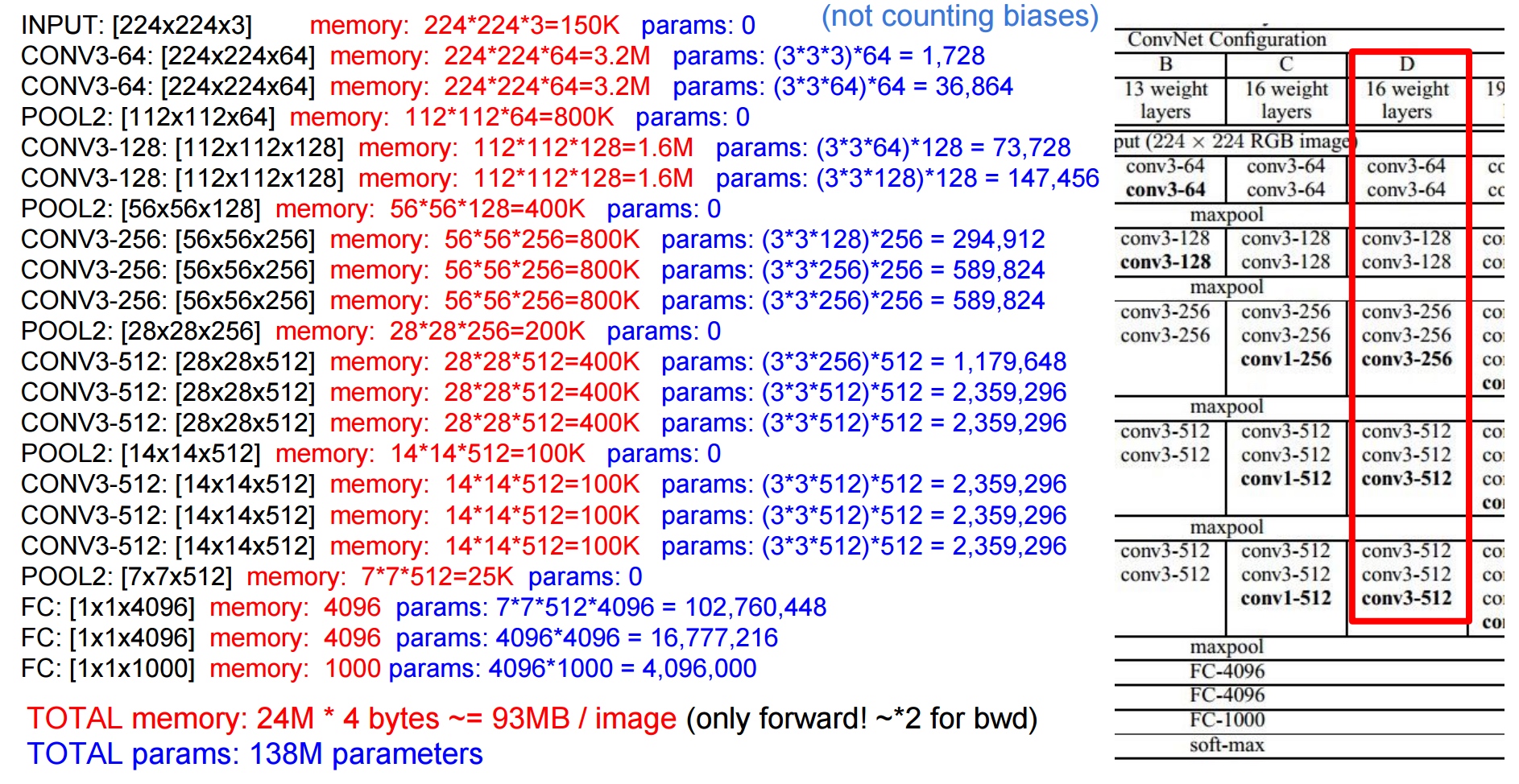
採用較小的Filter尺寸-3x3,卷積的間隔s=1:
1:3x3是最小的能夠捕獲上下左右和中心概念的尺寸。
2:兩個3x3的捲基層的有限感受野是5x5;三個3x3的感受野是7x7,可以替代大的filter尺寸。
3:多個3x3的捲基層比一個大尺寸filter卷基層有更多的非線性,使得判決函數更加具有判決性。
4:多個3x3的捲積層比一個大尺寸的filter有更少的參數,假設卷基層的輸入和輸出的特徵圖大小相同為C,那麼三個3x3的捲積層參數個數3x(3x3xCxC)=27CC;一個7x7的捲積層參數為49CC;所以可以把三個3x3的filter看成是一個7x7filter的分解(中間層有非線性的分解)。
也使用過1x1 filter:
作用是在不影響輸入輸出維數的情況下,對輸入進行線性形變,然後通過Relu進行非線性處理,增加網絡的非線性表達能力。
Max-Pooling:2x2,間隔s=2;
論文中解釋關於僅使用3x3 conv kernel,因為兩個3x3的conv kernel疊合的reception field等效於一個5x5 conv kernel(亦即每個pixel可以correlate到周圍的5x5個pixel), 而三個3x3則可以等效於一個7x7,但兩層3x3的參數量僅有一層5x5的(3x3x2)/(5x5) = 0.72倍,而三層3x3參數量是一層7x7的(3x3x3)/(7x7)=0.55倍,對應到的範圍等效並且可使得需參數量更少,並且疊越多層Conv+ReLU的特徵學習能力比單一層Conv+ReLU來的更好。
- Filter Size
相對於AlexNet 每個Stage僅含有一個Conv層, Filter 7x7, VGG每個Stage有2~4個Conv層,而Filter只有3x3, 原論文內”This can be seen as imposing a regularization on the 7 × 7 conv. filters, forcing them to have a decomposition through the 3 × 3 filters”
他說7x7 filter可以被分解成若干个3x3的filter的叠加。
類比一下n維空間的向量x,x的正交分解
x = x1(1, 0, 0, ….) + x2(0, 1, 0, …) + x3(0, 0, 1,…) + … + xn(0, 0, 0, …, 1)
每一組的每一層的filter被類比成n維歐幾里得空間的基底。
若VGG的一組含有3層3x3的filter,則我們則假設一個7x7的filter可以被分解成3種“正交”的3x3的filter。
作者原文:First, we incorporate three non-linearrectification layers instead of a single one, which makes the decision function more discriminative.Second, we decrease the number of parameters: assuming that both the input and the output of athree-layer 3 × 3 convolution stack has C channels, the stack is parametrised by 3 32C^2 = 27C^2weights; at the same time, a single 7 × 7 conv. layer would require 72C^2 = 49C^2
後來googleNet
將3×3的卷積分解成31和13的卷積,可以減少33%的計算量,如果將3×3分解為兩個2×2的,可以減少11%的計算量,而且利用非對稱卷積的效果還更好。
實踐表明,不要過早的使用這種分解操作,在特徵映射大小為(12〜20)之間,使用它,效果是比較好的。